Doctoral Candidate: Loreto Valentina Romero Ponce
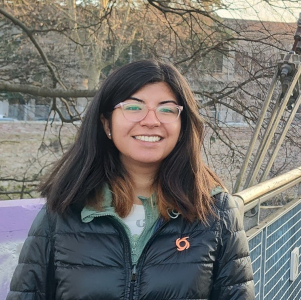
Host Institution: University of Padova (UniPD)
Project: Distributed characterization and sensing using HOM fibres
About
Loreto Valentina Romero Ponce studied Electronic Engineering at Universidad Técnica Federico Santa María (UTFSM) in Chile. Her specialization is in Telecommunications and she has specialized in Computer Networks and Optical Fibers Propagation. During the writing of her thesis at UTFSM, she implemented an interferometric optical phase detection scheme for Distributed Acoustic Sensors using Rayleigh backscattering, receiving her title in 2022.
In the last year, Loreto worked at a science and technology center from UTFSM where she led the commissioning and subsequent installation of a Chilean-designed equipment, capable of transmitting wave signals within the 5G-6G band, at the Nokia Bell Labs facility in Murray Hill, New Jersey, USA.
Project Objectives:
The main objective is to develop novel and effective models and data analysis algorithms to characterize the local properties of SDM fibres by analyzing the Rayleigh scattering of the fibre. This should be done having in mind both fibre distributed characterization and its application to distributed sensing. Concisely, the activity consists in the following main tasks:
- Implementation of an accurate numerical system model to generate realistic synthetic distributed measurements.
- Theoretical analysis of the intrinsic limits and bounds of distributed measurements.
- Design and development of novel algorithms to determine specific properties of the fibre, such as intrinsic modal dispersion and bending-and twist-induced coupling.
- Implementation of experimental testbeds.
- Experimental verification and application of the developed distributed measurement techniques.
Expected Results:
Exploring and exploiting use of multimode fibers to enhance performance of distributed sensors e.g. reflectometric measurements. Development of numerically assisted methods, using tools such as data-driven discovery of partial differential equations and machine learning (objective 3). To this aim, reliable synthetic data must be created (objective 1) and intrinsic bounds must be analyzed (objective 2). The techniques will be tested in controlled conditions (objective 4) and applied to various scenarios (objective 5).